If you asked me: “Do we need artificial intelligence (AI) to be better sport scientists?” I might retort by asking you in return: “Did we need electric guitars to make better music?”
I’ve spent the last ten years in the field of mathematical modelling. As a master-level student in mechatronics, I started out by modelling systems like electronic circuits, hydraulic circuits and multi-body systems. A few years later, I was fortunate to be enrolled in a PhD course in movement science at
CeRiSM Research Centre. That’s when I first came in contact with exercise physiologists and sport scientists, including work with lactate analysers and metabolimeters. I was amazed that sport scientists were, in fact, using the exact same equations I was using in my engineering courses. This meant that I could translate my expertise in hydraulics, electronics and mechanics to the modelling of physiological responses to cycling exercise, and actually contribute to the scientific community! What I love about modelling is that we can use our understanding of physics and physiology to describe the world we experience. And if we do a good job, the process of creating a model always leaves us with something to learn (4,6). Let me try to explain further.
In cycling, if we model the physiological response to exercise in terms of HR, VO
2 or [La] (see below), we are considering the metabolic control as a bit of a black box that is responding to the mechanical power requirements. In other words, we are assuming that there is no intelligence behind the control of the metabolic response. This is likely an assumption that most researchers involved in describing the metabolic control mechanisms would not like. However, when describing these responses, we can also observe parameters of the aerobic fitness that may change due to chronic adaptations to training (e.g. time-characteristic of the VO
2 kinetics (9) or lactate clearance ability (10)). Let’s assume that we could write a program that would suggest to us the type of training we should do to promote the optimal adaptations we need to enhance the various fitness parameters. In this case, we would be not only using computers to speed up the computations, but we could learn from this data and in return, perform more elaborate decision-making processes (6). In essence here, we would be creating an Artificial Intelligence (AI).
In the classic approach to AI, we create models using hand-written equations from physics and physiology. However, the modern approach to AI creation uses new technologies like neural networks (NN), machine learning and deep learning (8). The recent spread of user-friendly and proficient open source software, including
Tensorflow and
Keras (1), has opened the door for all of us to be able to implement machine learning in our own projects (e.g.
Kaggle competitions). What is really appealing of this modern approach, however, is that it does not require deep
a-priori knowledge about the system being modelled, nor extensive
a-priori knowledge on how to code an NN algorithm. I believe our dinosaur scientists out there won’t like the idea that just anyone now can rock up and write a physiological model without possessing any depth of understanding about the world of exercise physiology. But it’s equally true that without some level human expertise in the field, or understanding of sport
context, it will be impossible to interpret and apply the results where they count — in the real world of practice.
The recent proliferation of AI technologies is not only linked to the increased availability of open source machine learning software but equally to the explosion in data availability (e.g.
DimensionData). We are now living in a world that collects more data than it can actually process, and further gains in the future will come from enhanced efficiency in processing. A good analogy might be the field of video surveillance. Here we have the situation of near continuous data collection. Of course, however, if no one is watching the video, no one can spot the criminal in action. The job is simply too tedious. But if we could implement an AI substitute (12), we
could enhance the surveillance level and act more rapidly when the criminal activity takes place. To do this, we need to start slow and be patient with the process and let the AI learn from large numbers of examples (supervised learning). NNs can also work with un-labelled data (called unsupervised learning), but in most cases (e.g. contextualizing, dealing with unseen examples) supervised learning on labelled data works much better.
Now, what happens if our surveillance system fails? It’s a good question, and we should always weigh the pros and cons before applying any AI technology. Today, we’re perfectly comfortable in asking
Google or
Siri to play us a song that we’d like. We’re comfortable because we know that the worst case scenario is that Google Assistant plays us a song we don’t like. In more serious scenarios, if an AI commits a type-I error while raising the red flag for skin cancer from a picture (7), the worst that can happen is that the patient is examined one more time. If an AI surveillance system spots a criminal action that is not there, the worst that can happen is that the police will be sent for nothing. On the other hand, type-II errors from these scenarios may lead to more catastrophic consequences.
In the field of sport sciences, progress in AI is stimulating a more systematic approach to the areas of training monitoring and prescription. As athletes and coaches become more and more sceptical of seemingly ego-based decisions being made without sound rationale (5), they require methods that enable more data-based discussions permitting more coherent decisions to be made. Now, let’s assume that we want to create a modern AI virtual coach — one that exploits the power of NNs to tell us when to do HIIT, which of the 12 variables we need to manipulate to form the weapon, and eventually, the HIIT target type we are after, and when and how much to rest. This AI coach, before it can be used, needs to see probably hundreds of examples of training and resting periods, together with their resulting physiological responses (e.g., HR, VO
2, [La], session RPE, etc).
For example, in 2016, we (13) presented a similar tool: using an optimization algorithm and hand-written models to generate a large quantity of training sessions, before asking our AI to find the HIIT protocols that could maximize the time spent above 90% of VO
2max or to find protocols that maintained the highest level of [La] (i.e., type 1 vs 3 HIIT responses). The worst that could happen was that our prescribed HIIT protocol could not be sustained by our mock athlete, but the advantage was that we had the opportunity to run thousands of simulations to better evaluate the interplay between aerobic and anaerobic metabolic responses during different HIIT sessions. In 2017, at the SISMES National Conference (14), inspired by the work of Beltrame and co-workers (3), we presented an NN (Fig. 1) we could use to classify cycling intensity and VO
2 values (Fig. 2). In this case, the worst that could happen was that we wouldn’t estimate the energy expenditure of the training correctly.
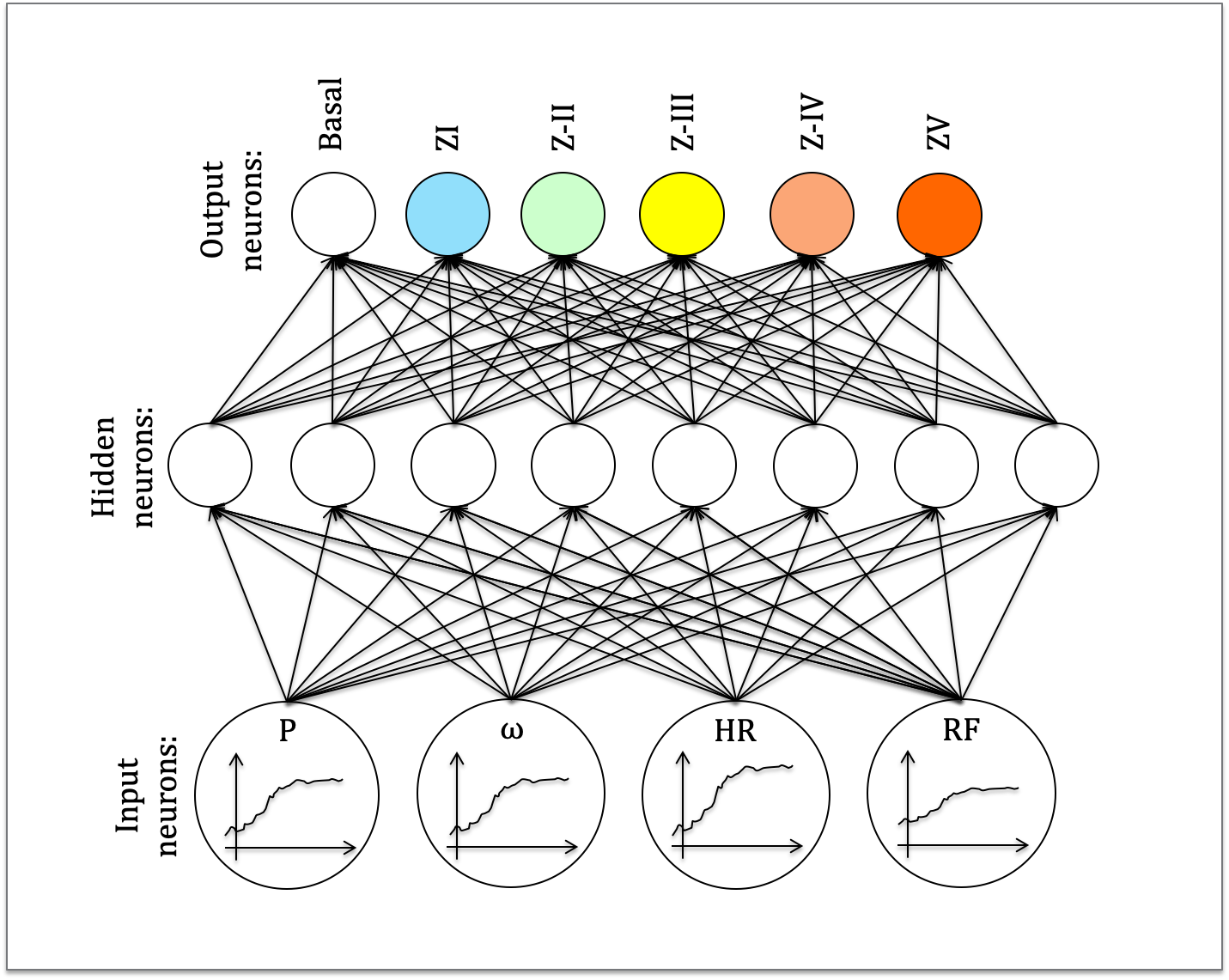
Fig. 1 This NN is a classifier designed to process easy-to-obtain inputs and to classify cycling intensity. Inputs like mechanical power (P), cadence (w), heart rate (HR) and respiratory frequency (RF) are processed by the NN that outputs the probability of the exercise intensity falling into different training zones (I-V). In this figure, a very generic architecture is shown: the number of hidden-layer and hidden-neurons can vary.
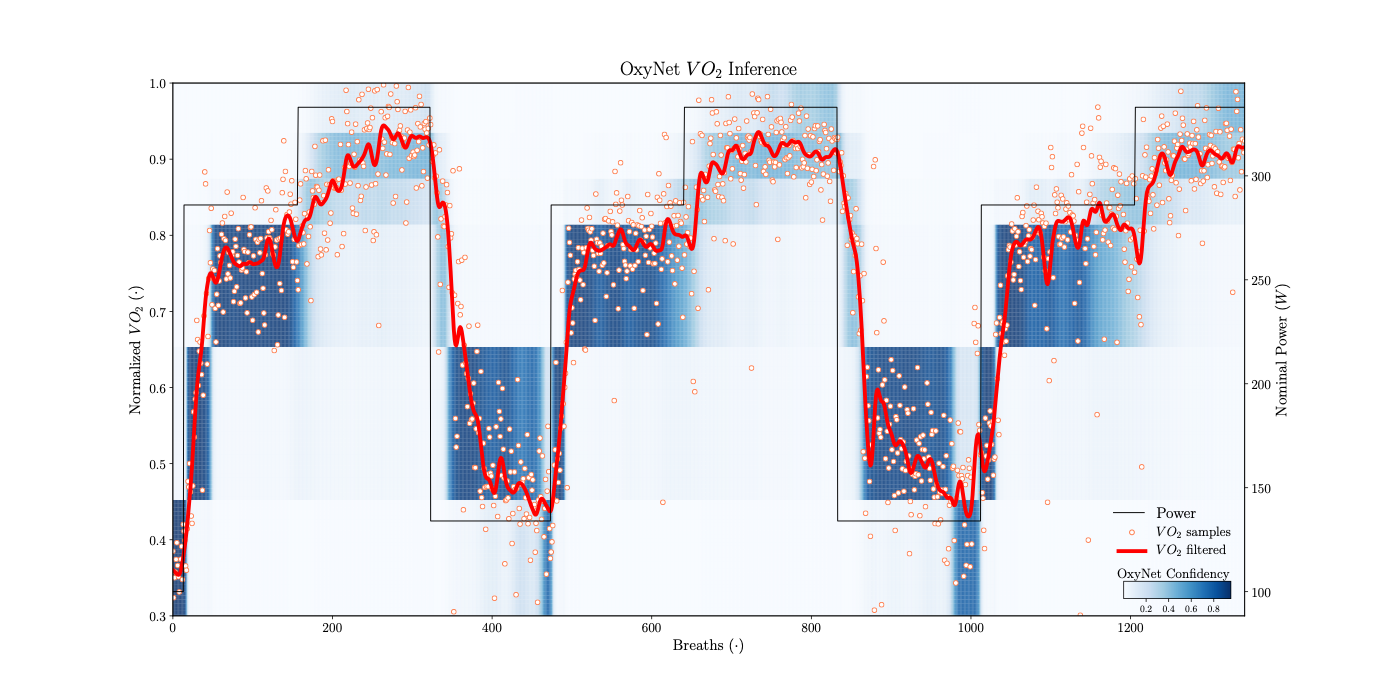
Fig. 2 With the NN presented in Fig. 1 we were able to predict complex traits of the VO2 response to exercise like slow component and post-exercise oxygen consumption excess. In this figure, the probability of VO2 of falling in one of the training zones is reported: the darker the blue colour, the more confidence the NN has in providing the training zone. The small dots and the continuous line represent the experimental raw and averaged VO2 signals respectively. This NN can work on a real-time basis (breath-by-breath).
The worlds of sport sciences and high performance sport are meritocratic. So if decisions from an assistant AI coach will not benefit the coach or athlete, then adoption won’t occur. However, I feel we have only just started scratching the surface (2) and the full potential of AI has yet to be tested, explored and deeply discussed. Whether it will be great or not actually doesn’t matter to me, because today it scratches a real itch. Just like the training program puzzle we all strive to solve with HIIT, putting AI technologies in the position where they may leverage our ability to solve the puzzle, is in itself my puzzle.
When we look to history, it’s apparent that new methods are often celebrated too fast, while old methods can be promptly dismissed and replaced. On the other hand, it often takes many years for methods to be accepted and become mainstream. Before celebrating, dismissing or vilifying any methodology, we need a regular process of intentions, discussions and rational-based conclusions. Especially in sports, there will always be something that cannot be counted or expressed in numbers. That’s the magic of working with athletes in the first place. Along these lines, I’m simply not afraid of what AI will be able to do or not to do, because I don’t think it will change our world to the point where there won’t be a veil of magic anymore. I believe that the veil of magic will resist the test of time, including the test of new AI technologies. My only trepidation is that we might not be ready to carry out these tests with the scientific rigour they deserve (11).
In conclusion, I don’t believe that we need AI to become better coaches or better sport scientists. However, we need AI to test old ideas, and form new ones, to stimulate debate, and move our optimization forward. That is all every technology is about. AI, like all technologies, can be used, abused and misused. However, as with each technology or tool, it brings us the
chance to test our convictions and change our positions. It aids us to refine the scientific process.
So, back to your first question: “Do we need AI to be better coaches or sport scientists?” My answer today would be: “No, I don’t think so”. We didn’t need electric guitars to make better music, and we definitely don’t need AI to become better coaches or sport scientists. We needed electric guitars to create new sounds, stimulate new ideas, evolve new melodies and make songs for the new generations. AI as applied to coaching and sport science will be similar.
Author’s bio: Andrea Zignoli is currently holding a post-doc position at the Department of Industrial Engineering of the University of Trento, where he develops mathematical models for knee biomechanics and cycling physiology. You can follow updates on his research on
ResearchGate,
LinkedIn, and
Twitter (@andrea_zignoli).
References
- Abadi, M, Agarwal, A, Barham, P, Brevdo, E, Chen, Z, Citro, C, et al. Tensorflow: Large-scale machine learning on heterogeneous distributed systems. ArXiv Prepr ArXiv160304467 , 2016.
- Allen, SV and Hopkins, WG. Big Data and More at the 2017 Sports Analytics Conference, Australia. Sportscience 23–26, 2017.
- Beltrame, T, Amelard, R, Villar, R, Shafiee, MJ, Wong, A, and Hughson, RL. Estimating oxygen uptake and energy expenditure during treadmill walking by neural network analysis of easy-to-obtain inputs. J Appl Physiol 121: 1226–1233, 2016.
- Beneke, R. Experiment and computer-aided simulation: complementary tools to understand exercise metabolism. Biochem Soc Trans 31: 1263–1266, 2003.
- Buchheit, M. Outside the Box. Int J Sports Physiol Perform 12: 1001–1002, 2017.
- Clarke, DC and Skiba, PF. Rationale and resources for teaching the mathematical modeling of athletic training and performance. Adv Physiol Educ 37: 134–152, 2013.
- Esteva, A, Kuprel, B, Novoa, RA, Ko, J, Swetter, SM, Blau, HM, et al. Dermatologist-level classification of skin cancer with deep neural networks. Nature 542: 115–118, 2017.
- Goodfellow, I, Bengio, Y, and Courville, A. Deep learning. MIT press, 2016.
- Jones, AM and Koppo, K. Effect of training on VO2 kinetics and performance. In: Oxygen uptake kinetics in sport, exercise and medicine.Routledge, 2005. pp. 373–398
- Messonnier, L, Freund, H, Denis, C, Féasson, L, and Lacour, J. Effects of training on lactate kinetics parameters and their influence on short high-intensity exercise performance. Int J Sports Med 27: 60–66, 2006.
- Peake, J, Kerr, GK, and Sullivan, JP. A critical review of consumer wearables, mobile applications and equipment for providing biofeedback, monitoring stress and sleep in physically active populations. Front Physiol , 2018.
- Russell, S, Dewey, D, and Tegmark, M. Research priorities for robust and beneficial artificial intelligence. Ai Mag 36: 105–114, 2015.
- Zignoli, A, Fornasiero, A, Savoldelli, A, Morelli, M, Bertolazzi, E, Biral, F, et al. An optimal control approach to the high intensity interval training design. J Sci Cycl 5, 2016.
- Sismes IX National Congress: Brescia, September 29–October 1 2017. Sport Sci Health 13: 1–102, 2017.