Background
High-Intensity Interval Training (HIIT) is a time-efficient approach to maximize physiological adaptation and optimize sports performance. In 2013, HIITscience founders Martin Buchheit and Paul Laursen wrote a review called “High-Intensity Interval Training, Solutions to the Programming Puzzle”. Indeed, prescribing HIIT sessions can be puzzling because they are composed of many parameters that impact the sessions’ level of difficulty and the resulting physiological adaptations.
As an international level triathlete competing for Canada, I performed thousands of HIIT sessions over the years. I was introduced to HIIT modeling in 2019, just after completing my B.Sc in physics and mathematics. I joined a project (which would become my MSc. project), under the supervision of Guy Thibault and Jonathan Tremblay at the University of Montreal and the Institut National du Sport du Québec, aiming to build a web app helping practitioners solve the HIIT programming puzzle according to their athletes’ profile and objectives.
The first natural step was to assess two popular models of HIIT programming, the Skiba and Coggan models. The Skiba model is widely referred to in the scientific literature. Based on the Monod and Scherer continuous exercise hyperbolic model, it enables the calculation of the critical power (CP) and an anaerobic reserve (W’). The CP is described as a steady-state work boundary. Work intensities performed above the CP deplete the W’, while work intensities performed below the CP replete the W’. Once the athlete’s W’ is fully depleted, the athlete is assumed to be exhausted. The Coggan model constitutes the foundation of the metrics used in the popular application TrainingPeaks (https://www.trainingpeaks.com/). It introduces new metrics: the normalised power (PN), intensity factor (IF) and training stress score (TSS). These metrics are meant to better represent the curvilinear relationship between the exercise power output and physiological stress.
While most of the critics on the Skiba model concern either the W’ replenishment calculations or the physiological interpretation of the CP, we were wondering how the Monod and Scherrer model limitations in the heavy and severe intensity domains propagated to the Skiba model. In addition, the models essentially rely on metrics such as the CP, W’, and FTP. Two athletes with the same FTP could have different performances on longer or shorter effort durations. We wanted to verify if the models could be quickly adapted to account for the athletes global performance profile. To answer these questions, we started by exploring the limitations of the Skiba model and the Coggan model to see how they could potentially affect the practitioners prescription and analysis of HIIT sessions.
What we did?
Obtaining quality HIIT data from a large group of subjects being very difficult, we opted to proceed with simulations. We simulated three fictitious athletes profiles: a Sprinter, performing better on short duration efforts, an All-Rounder, displaying average performance level on any duration, and a Time-Trialist, performing better on longer effort duration. Table 1 presents the power output of each fictitious athlete over a range of durations.
Performance duration | Sprinter (W) | All-Rounder (W) | Time-Trialist (W) |
1 s | 1251 | 1115 | 978 |
15 s | 968 | 876 | 777 |
30 s | 782 | 719 | 647 |
45 s | 665 | 623 | 567 |
1 min | 589 | 561 | 516 |
2 min | 457 | 454 | 431 |
3 min | 411 | 419 | 403 |
4 min | 389 | 402 | 390 |
5 min | 375 | 391 | 382 |
10 min | 317 | 341 | 342 |
20 min | 276 | 307 | 315 |
30 min | 257 | 290 | 301 |
45 min | 239 | 274 | 289 |
60 min | 227 | 264 | 281 |
90 min | 211 | 249 | 269 |
2 h | 199 | 239 | 261 |
4 h | 172 | 214 | 241 |
A wide range of HIIT sessions were simulated by varying the interval duration and rest durations between 15 s and 5 min by increments of 15 s, and the number of repetitions between 2 and 20 by increments of 1 repetition. By doing so, we obtained 6198 combinations of parameters. We arbitrarily set the sessions’ rest intensity at 50% of the athletes’ maximal aerobic power (MAP), which we defined as the maximal power output over 5 min. For each of these numerous sessions, we calculated what power output the fictitious cyclists would have to perform the effort intervals at so that they would theoretically (according to each model) be exhausted at the end of the last repetition. Note that we had to modify the Coggan model (Coggan-Modified model), by introducing the notion of exhaustion, which was not well represented in the original model. The Coggan-Modified compares the normalized power of the HIIT session with the athletes’ record power output over the sessions’ duration and, therefore, takes into account the athletes’ global performance profile. In the end, we obtained three sets of 6198 HIIT sessions leading to exhaustion (one for each fictitious athlete), for both models (Skiba and Coggan).
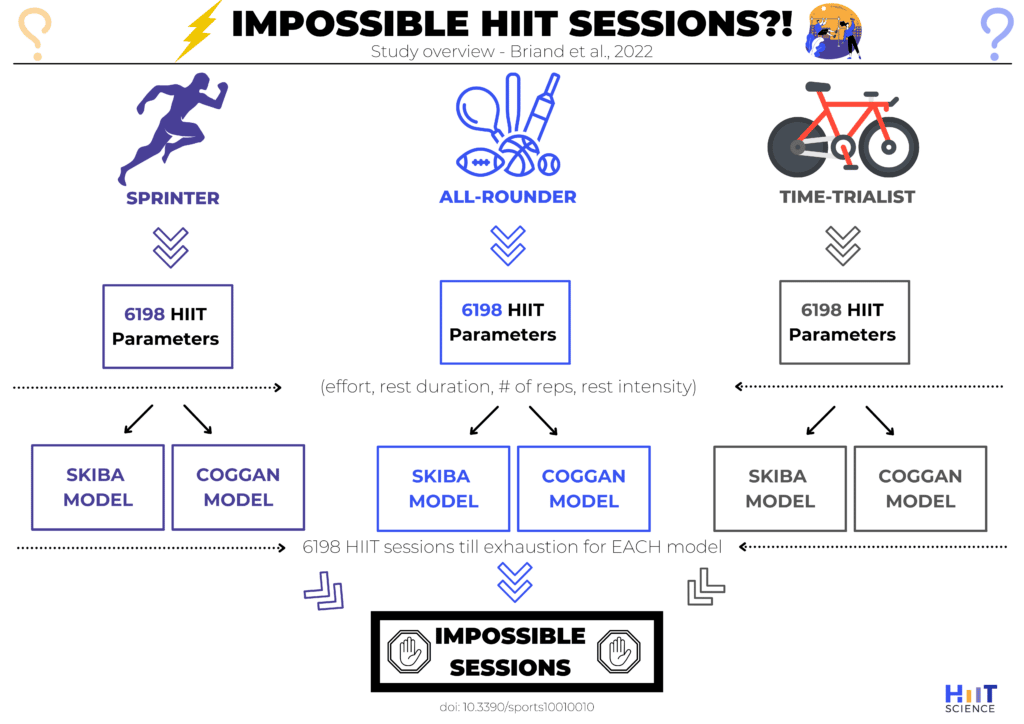
What we found?
As depicted in the figure, for each of the two models and each athlete profile, we found many sessions that would be impossible to execute in practice. Impossible sessions occur when the cyclists would have to perform every effort interval of the session at an intensity higher than their record power output over the interval duration.
The proportion of impossible sessions was always higher for the Skiba model and tended to be higher for athletes with greater endurance and lower anaerobic capacities. The impossible HIIT sessions were characterized by a low number of short effort intervals, performed within the severe or extreme intensity domains, interspersed with a long recovery. The impossible sessions are mainly due to mathematical assumptions made by Skiba and Coggan when they elaborated their models.
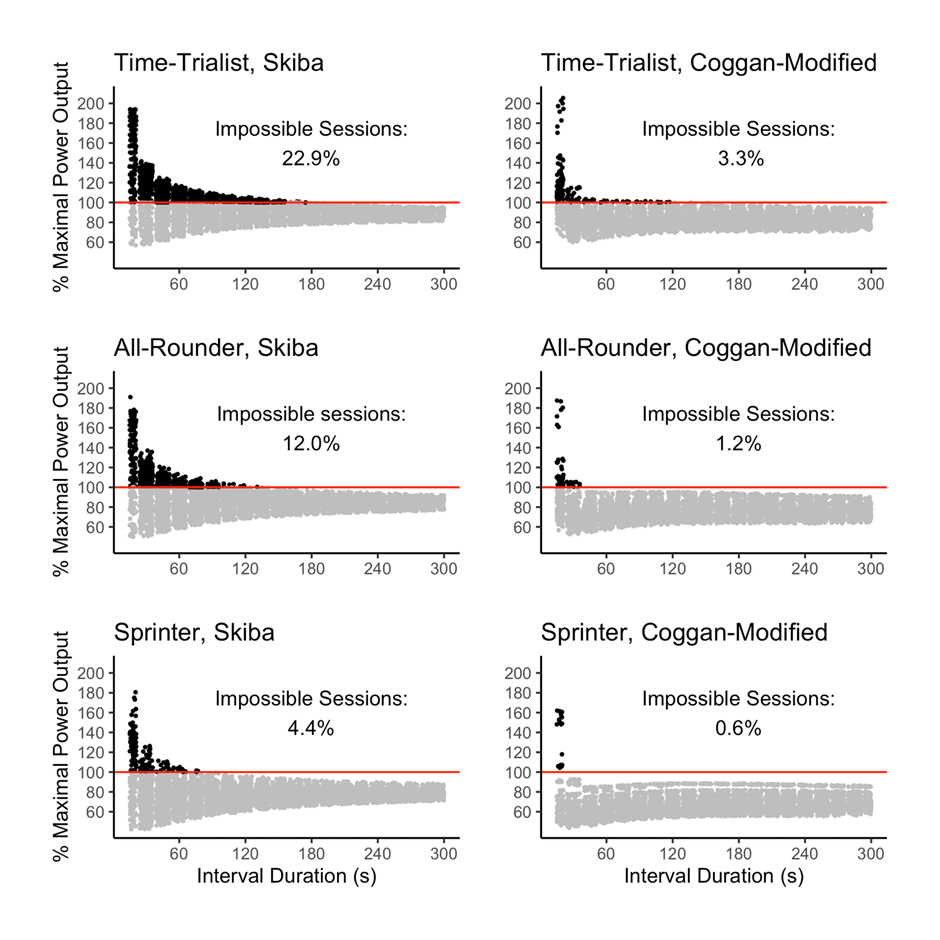
Practical Applications
HIIT sessions performed in the moderate intensity domain (between ~ 85 to 100% of MAP) are traditionally very popular in cycling. Both models (Skiba and Coggan-Modified) seem adapted to prescribe HIIT sessions in that intensity regime. Our simulations do not highlight impossible sessions specifically in the moderate intensity domain. However, training methods keep evolving. Novel training approaches, such as sprint interval training (SIT) and polarised training are oriented towards the severe and extreme intensity domains. They bring different physiological adaptations that could potentially lead to similar or even improved performances compared to traditional approaches. The Skiba and Coggan models are also used to analyze, predict and potentially manage performance in competition, which very often include short bursts of high intensity efforts. Using any of the two models to prescribe, manage or analyze SIT, polarised training or even racing performances could lead to wrong conclusions because of the models’ mathematical limitations.
We encourage practitioners to be aware of how both models’ mathematical assumptions influence the models’ prescription and analysis in the specific context they are to be used. To individualize prescription and analysis, the athletes’ global performance profile should be used rather than solely performance metrics such as the CP, W’ or FTP. No model is perfect, but, in that sense, the Coggan-Modified model remains less limited than the Skiba model.
The next step in our research at the University of Montreal and the Institut National du Sport du Québec will be to develop a more appropriate physiological model of HIIT. Our ultimate goal is to provide a tool that allows practitioners to solve the HIIT programming puzzle according to the athletes’ profile and goals.
About the authors
Jérémy Briand: jeremy.briand@umontreal.ca
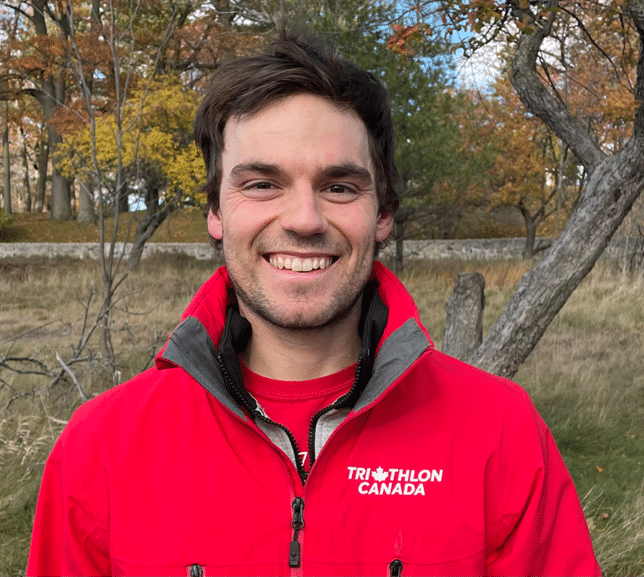
Jérémy is currently finishing his MSc on HIIT modeling at the University of Montreal. In 2019 he obtained his BSc in physics with a specialization in mathematics at McGill University. He also works as a Research Assistant at the Institut National du Sport du Québec. He is an international level triathlete, still competing for Canada on the World Cup and World Triathlon Series circuits. In his research, Jérémy is looking to join his high performance sports experience to his physics and mathematics knowledge in order to provide more efficient tools for sport practitioners that help athletes reach their full potential.
Guy Thibault: gthibault@insquebec.org
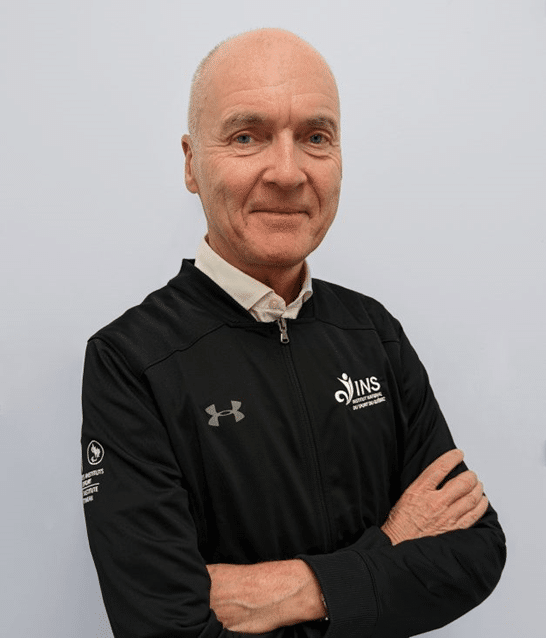
Dr. Guy Thibault is the Sport Science Director at the Institut National du Sport, while being an affiliated Professor at the University of Montreal. He is one of the masterminds behind Canada’s recent success at the Summer and Winter and Olympic Games. Having written multiple books on endurance sports, he has mastered the art of making scientific knowledge accessible to sport practitioners and the general public. His main research interest revolves around understanding HIIT and how to make it an even more efficient training method. Guy spends most of his spare time on his bike (either outside or on Zwift), experimenting new HIIT methods.
Jonathan Tremblay: jonathan.tremblay@umontreal.ca
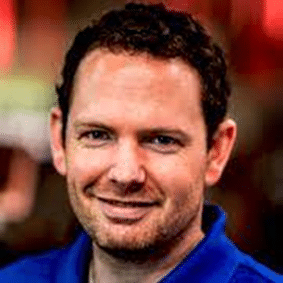
Dr. Jonathan Tremblay is an Associate Professor at the “École de kinésiologie et de l’activité physique (EKSAP)” at the University of Montreal. His current research work explores the effects of maple product ingestion during prolonged exercise on performance and cognitive function, the metabolic responses and chronic adaptations of eccentric cycling training, training load monitoring associations with performance and injuries, applications of machine learning to sports, as well as HIIT modeling. Until 2017, he was Sport Science Director at the Institut National du Sport du Québec. Having worked with high level athletes in the past, he is constantly looking at ways to integrate his research findings to optimize athletes’ performance.